How to Tame Your Raspberry Pi's Local AI
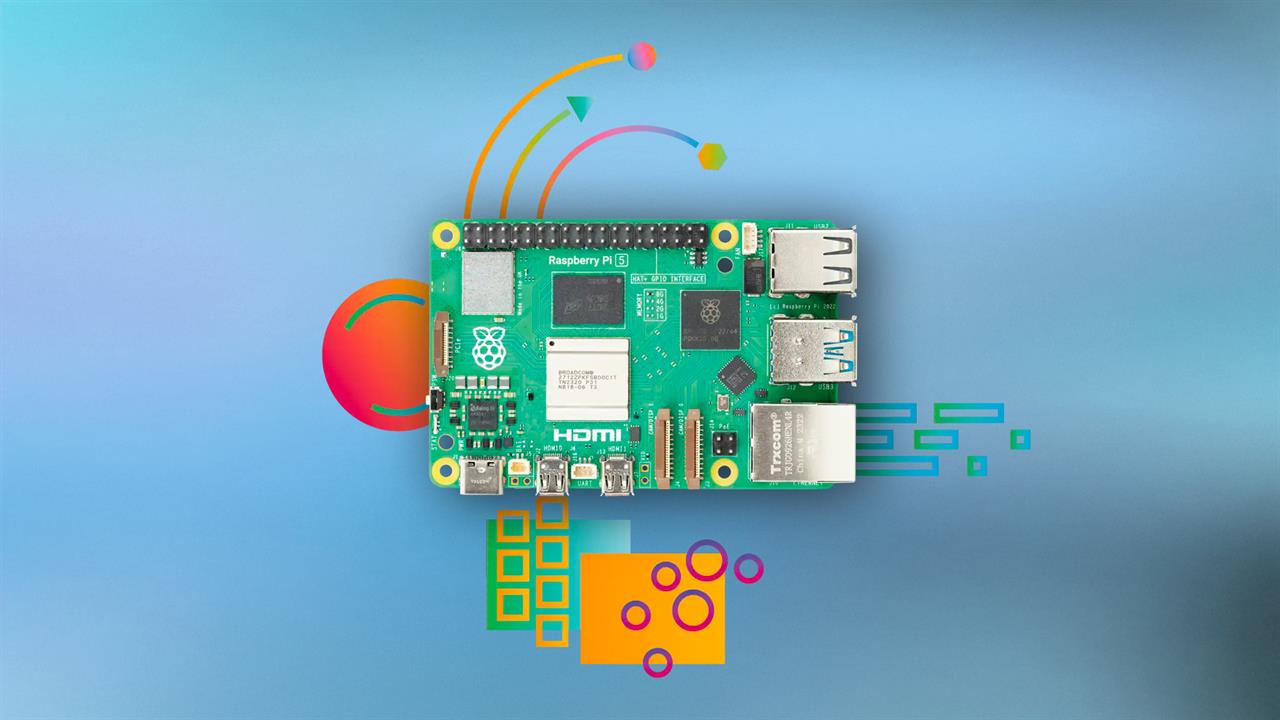
This is part two of a tutorial series on building an AI voice assistant that runs locally on Raspberry Pi hardware. You can read part one here.
In the first part of this project, we showed you how to build an AI voice assistant on a Raspberry Pi using a combination of OpenvoiceOS and Ollama. But we're not done yet.
The problem is that the AI part of my voice assistant Boris takes ages to respond, because the Raspberry Pi only has a limited amount of computing power, and the Large Language Model (LLM) Boris is running requires significant amounts of memory and processing power to run.
Read more: How To Create an AI Voice Assistant with Raspberry Pi
The result? Boris often takes 15 to 30 seconds to respond to a question, because the limited memory and processor power of the Pi 5 limits the speed it runs at. Generally speaking, you measure the speed of an LLM in tokens per second (TPS), which indicates how many of these fundamental units the system can process. For reference, Ollama tells me that my Pi 5 running Phi 3 can manage about 3 TPS.
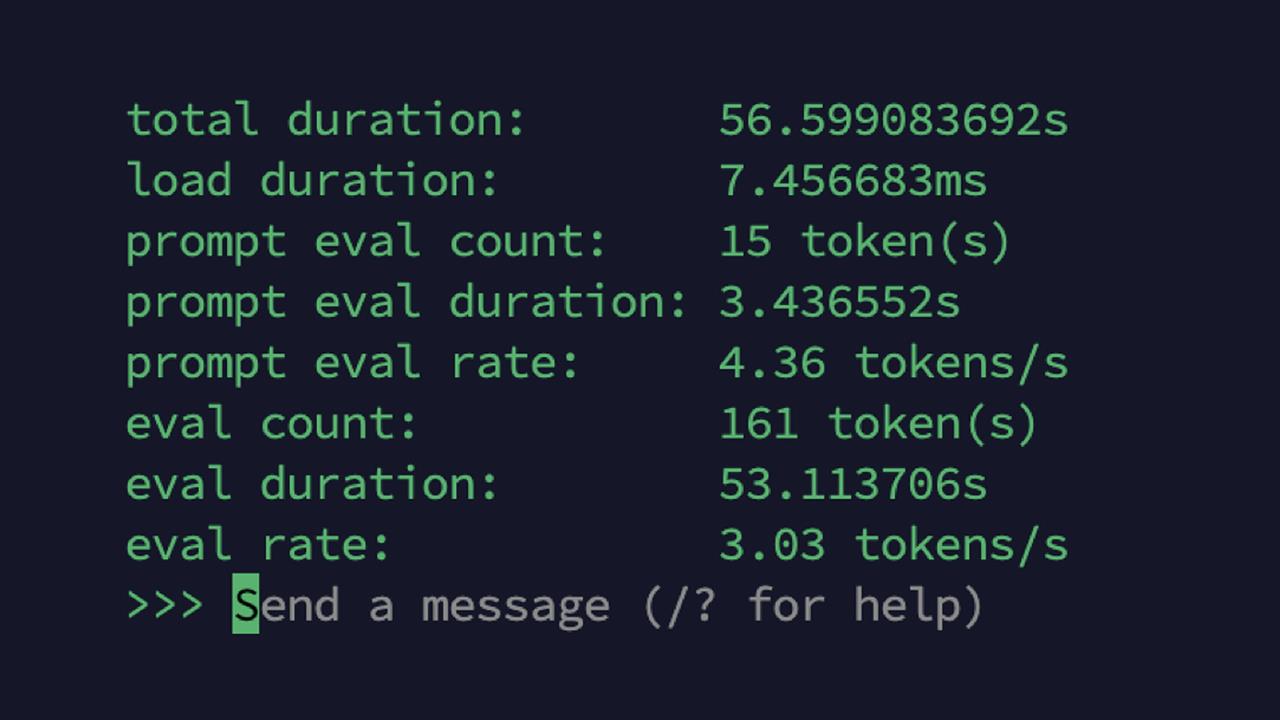
I use the Phi3 LLM created by Microsoft, which is built to run on devices like phones, which will have a Graphics Processing Unit (GPU) or a Neural Processing Unit (NPU), a dedicated chip that is great at throwing around large chunks of data. That’s what LLMs are built on, and modern phones will probably have one or both, while the Pi 5 has neither.
Fortunately, there are a few ways that you can make things work a bit quicker, without undermining our aim of keeping your data private and local. I’ll show you four ways: adding an NPU, tweaking the model parameters, quantizing, and adding more computing power by distributing the load.
Add an NPU
The Raspberry Pi 5 does not come with an NPU, but you can add one. Several are available, with the official Raspberry Pi AI Kit for about $70, which adds a Hailio-8L, which they call an “AI Accelerator.” However, this is more focused on image processing, so it might not make much difference on our LLM. (It is technically possible to add a GPU to a Pi 5, but it’s a complex process and rather defeats the purpose of having a small, simple computer.)
Customizing the LLM
Ollama has the ability to easily customize LLMs: you simply create a new modelfile, a text file that includes parameters to control how the model is used. After some experimentation with Boris, I came up with this modelfile, which I saved as Phi-Boris.txt:
FROM phi3:latest
SYSTEM You Are Boris, a helpful AI Assistant who answers in under 15 words.
PARAMETER temperature 0.9
PARAMETER top_k 40
PARAMETER top_p 0.7
I then created a new LLM by running this command on the Pi:
Ollama create borisphi -f /Phi-Boris.txt
That takes the Phi3 LLM, adds on my parameters and saves the LLM. I can then run it the same way as any other LLM:
Ollama run Phi-Boris
The tweaks made it more focused on working as a voice assistant. It doesn’t change the fundamental speed of the thing, though: the new LLM has the same TPS speed as the old one, but the shorter answers are quicker to produce.
Use a Quantized Model
Think of a LLM as a huge list of probabilities of what word comes next: if the first word is “who,” there is a certain probability that the next word will be “is,” and another probability that it will be “put.”
If we have a specific purpose that requires a specific list of words, we could make the list shorter and quicker to read by only including the words we need. That’s a process called quantization, which makes the model more efficient and thus faster for the specific task. It also makes it less accurate because the shorter list may miss options, So it’s a balancing act, but it is worth it if you are aiming for a specific purpose, such as a voice assistant to answer general queries.
Doing it yourself is possible, but it is a very complex task. Fortunately, like most things in AI, someone else has usually already done the hard work for you. After some poking around I found this quantized version of Phi3 that was tweaked to run on a CPU-only platform like the Pi 5. It is also published on the Ollama models list, which makes it immeasurably easier to install with a single command:
ollama run jpacifico/chocolatine-3b
Running this LLM, my Pi 5 managed about 3.1 TPS. That’s a minor improvement, but every little bit helps. I also used the customizing technique above to make it more focused on the short, snappy responses I seek.
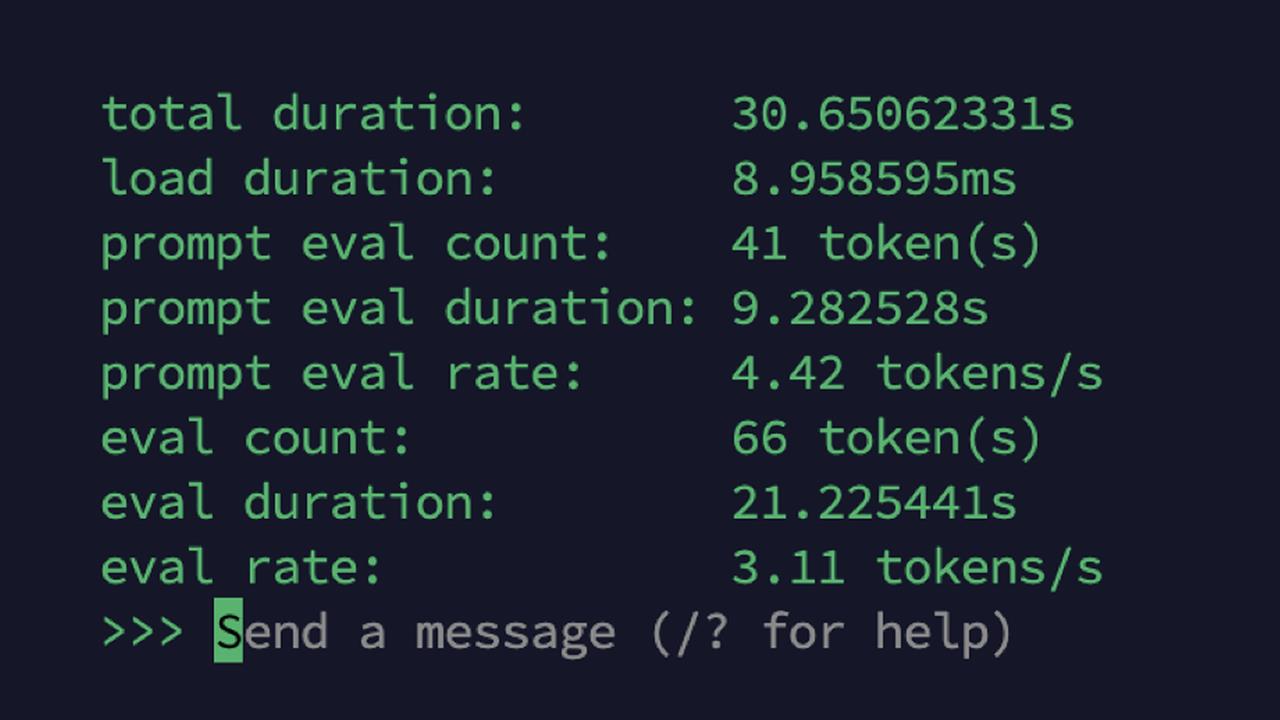
Screenshot: Richard Baguley
However, this does show that there isn’t much we can do to make it quicker. However much we tweak and configure the LLM, it will always be slow on a Pi. Fortunately, we can step beyond that and add in much more computing power.
Run Ollama On A Faster Computer
Need to crack a nut quicker? Use a bigger nutcracker. Ollama has a built-in server that allows you to call it from over a network, so the easiest way to make it faster was to run it on a faster computer. On my M1 Mac Mini, Ollama could crank through a much more respectable 22 TPS. On a recent Dell laptop with an NPU in the Intel CPU, I got 53 TPS: over ten times faster than the Pi. To set the Pi to access this computer, I simply made this change to the settings file for OVOS that I described in my last piece:
{
"persona": "You are a helpful voice assistant.",
"__mycroft_skill_firstrun": false,
"enable_memory": true,
"memory_size": 10,
"name": "my brain",
"confirmation": false,
"api_url": "http://MACHINENAME:11434/v1",
"key": "sk-xxx",
"model": "boris-phi"
}
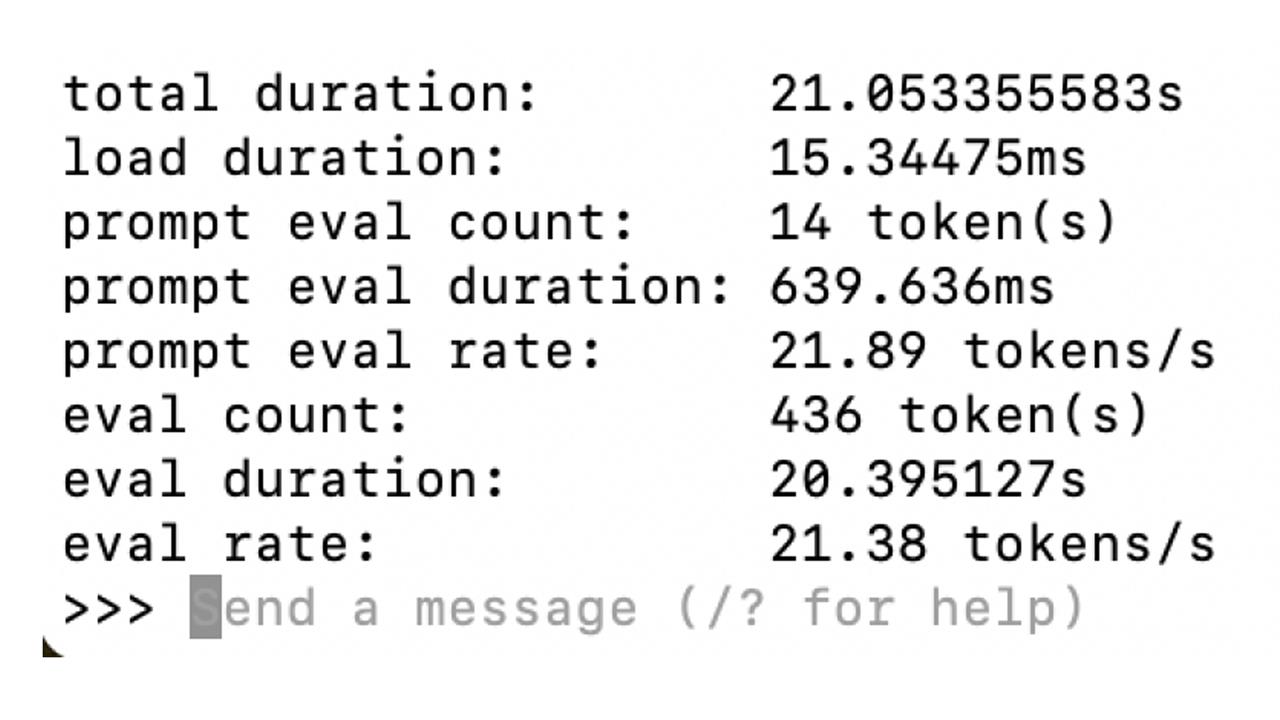
Screenshot: Richard Baguley
Replace MACHINENAME with the IP address of the remote computer, and it will use it for AI questions. This isn’t restricted to my local network, though: if I wanted to, I could run Ollama in the cloud or spin up a virtual computer using a service like Paperspace. But I'm also able to choose to keep everything on my home network, and away from any computers not under my direct control.
So where are we now with Boris? Boris can answer simple questions quickly, can use a local AI for trickier ones, and can tap into other remote resources like Wolfram Alpha when required. That’s a great start, but I think Boris is just getting started, so stay tuned for more.
Read more: AI Tools and Tips
- How to Use an AI Agent
- DeepSeek's New AI Challenges ChatGPT — and You Can Run It on Your PC
- How To Improve Your AI Chatbot Prompts
- How I Turned Myself into an AI Video Clone for Under $50
- What is Meta AI? A Capable Chatbot That’s 100% Free
- How to Get Started with Copilot for Microsoft 365
- Getting started with LM Studio: A Beginner's Guide
- Meet Claude, the Best AI You've Never Heard of
Richard Baguley is a seasoned technology journalist and editor passionate about unraveling the complexities of the digital world. With over three decades of experience, he has established himself as a leading authority on consumer electronics, emerging technologies, and the intersection of tech and society. Richard has contributed to numerous prestigious publications, including PC World, Wired, CNET, T3, Fast Company and many others.